ONNX Model format in Flink
Alexey Novakov published on
7 min, 1352 words
The most popular eco-system to train ML model these days is Python and C/C++ based libraries. An example of model training can be a Logistic Regression algorithms, from ScikitLearn package or more advanced neural networks algorithms offered by Tensorflow, Pytorch and others. There are lots of tools and libraries in Python world to facilitate training and model serving.
In order to bring trained models in Keras or SciKitLean into a Flink application, we can use cross-platform file formats such as ONNX and PMML (in the past). These formats also come with language runtimes. In Flink case, we select JVM SDK to run inference logic inside the Flink job.
Let's look at the example on how to train Logistic Regression in Python using Keras and then use trained model in ONNX format inside Flink.
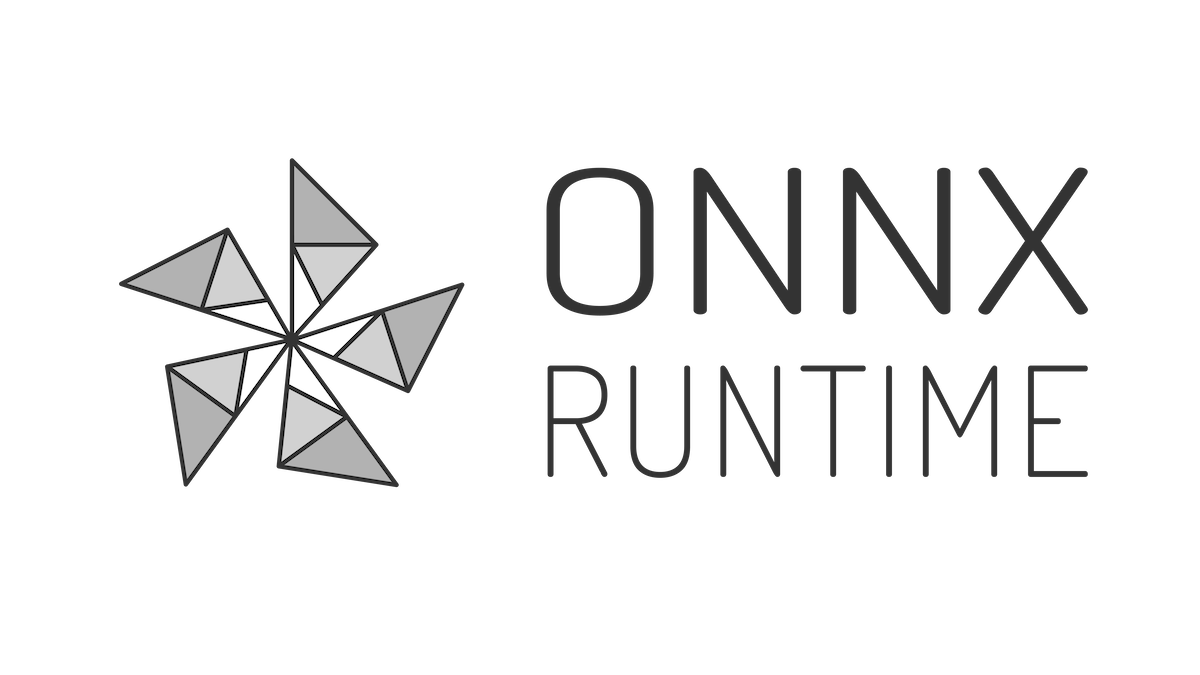
Read More
Categories: scala
Tags: flink machine learning scala tensorflow onnx